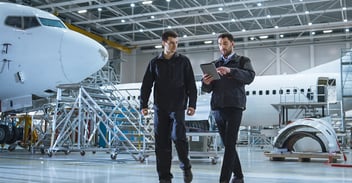
Overcoming Challenges in Applying AI/ML for Predictive Maintenance in Aviation MRO
Published :
The aviation Maintenance, Repair, and Overhaul (MRO) industry is on the brink of a technological revolution, driven by advancements in artificial intelligence (AI) and machine learning (ML) in MRO software. The global AI in aviation market is projected to exceed $9 billion by 2030 from $653.74 million in 2021, with a CAGR of 35.38% from 2022 to 2030.
Some examples of the use of AI in MRO are as follows:
However, despite the immense potential, implementing successful predictive maintenance models using AI/ML in MRO presents several challenges.
While the benefits are clear, the path to successful implementation is difficult. Some of the significant challenges are:
Predictive maintenance models in MRO aviation software require large datasets with high-quality, consistent data to train effectively. However, data in the aviation industry is often siloed, inconsistent, and lacking in standardization.
Many MRO organizations still rely on legacy systems not designed to handle the advanced capabilities of AI/ML. This integration requires substantial investment in advanced MRO software and training for the workforce, which can be a significant barrier for many organizations.
The aviation industry is highly regulated, and any new technology, including MRO aviation software, or process must comply with stringent safety and operational standards. Ensuring that AI/ML models meet these standards can be complex and time-consuming.
Dr. Yvonne Cagle, an experienced aerospace medical doctor, points out, "Data used to train AI/ML models must be comprehensive and of high quality. Inconsistent data leads to inaccurate predictions, undermining the trust in these systems."
Mark Rogers, a leading data scientist in the field, emphasizes the importance of workforce readiness, "Successful implementation of predictive maintenance requires not just advanced technology, but also a workforce that understands and trusts these new tools. Continuous training and education are essential to bridge this gap."
To overcome these challenges, industry leaders suggest a phased approach to implementation: Start with pilot projects that allow for the testing and refinement of predictive models in MRO aviation software on a smaller scale before a full-scale rollout. This approach helps in identifying and addressing potential issues early on.
Monica Badra, Founder of Aero NextGen, underscores the importance of collaboration. "Collaboration between MRO aviation software providers, technology vendors, and regulatory bodies is crucial. Working together, we can ensure that AI/ML models are not only effective but also compliant with industry standards," she adds.
Manoj Singh, President of Global Aerospace, Aviation, and Defense at Ramco Systems, adds, "Our Aviation ERP solutions are designed to integrate seamlessly with AI/ML technologies, providing a robust platform for predictive maintenance. By leveraging our comprehensive data management capabilities, MROs can ensure that their predictive models are trained on high-quality, consistent data."
While the road to successful implementation of predictive maintenance models in aviation MRO is challenging, the potential benefits make it a worthwhile endeavor. By addressing data quality issues, investing in workforce training, and ensuring regulatory compliance, the industry can harness the power of AI/ML in MRO software to transform maintenance practices.
Through strategic collaboration and phased implementation, the vision of a predictive maintenance model that enhances safety, reduces costs, and improves operational efficiency can become a reality. As the aviation industry evolves, embracing these technological advancements in MRO software will be key to staying ahead in a competitive and rapidly changing landscape.
All Rights Reserved. © Copyright 2024. Ramco Systems.